Machine Learning for Embedded Systems with AIfES®
With the open source AI software framework AIfES (Artificial Intelligence for Embedded Systems) you can run and even train artificial neural networks (ANN) on virtually any hardware. Tiny embedded systems plus artificial intelligence (AI) - the topic of our time.
Open Source AI Framework
The first open source AI framework "Made in Germany", developed as a Maker project at the Fraunhofer Institute for Microelectronic Circuits and Systems IMS. AIfES® is comparable and compatible with well-known Python ML frameworks like TensorFlow, Keras or PyTorch. In the current version, Feedforward Neural Networks (FNN) and Convolutional Neural Networks (ConvNet) are supported, which can be configured completely freely. Common activation functions such as ReLU, Sigmoid or Softmax are also already integrated.
Tiny ML
AIfES® can be used on almost any system, be it a microcontroller, IoT device, Raspberry PI, PC or smartphone, making the purchase of new hardware redundant. However, the focus is particularly on running AI on simple microcontrollers and small IoT devices, so-called »tinyML«. Tiny, self-learning, battery-powered devices can process sensor data where it occurs, independent of a cloud or other devices. The data is stored on the device and processing takes place without transmission delay, with significantly lower energy consumption compared to a PC.
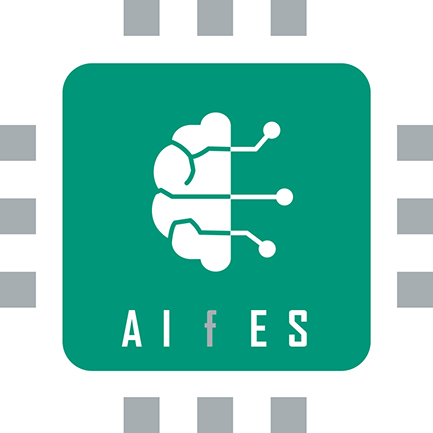