Faster and data reduced solution for high-resolution LiDAR systems
TimestampsAI is our development for your applications to capture complex scenes in 3D in any environment.
Distance determination method timestampsAI
Our distance determination method TimestampsAI bypasses data-driven problems for all future LiDAR sensor solutions. A pixel-wise quality information given in addition to the resulting point cloud allows confident decision-making of automated systems.
With the compact feature extraction and machine learning algorithms directly based on LiDAR timestamps, future LiDAR systems can be more efficient, effective and robust.
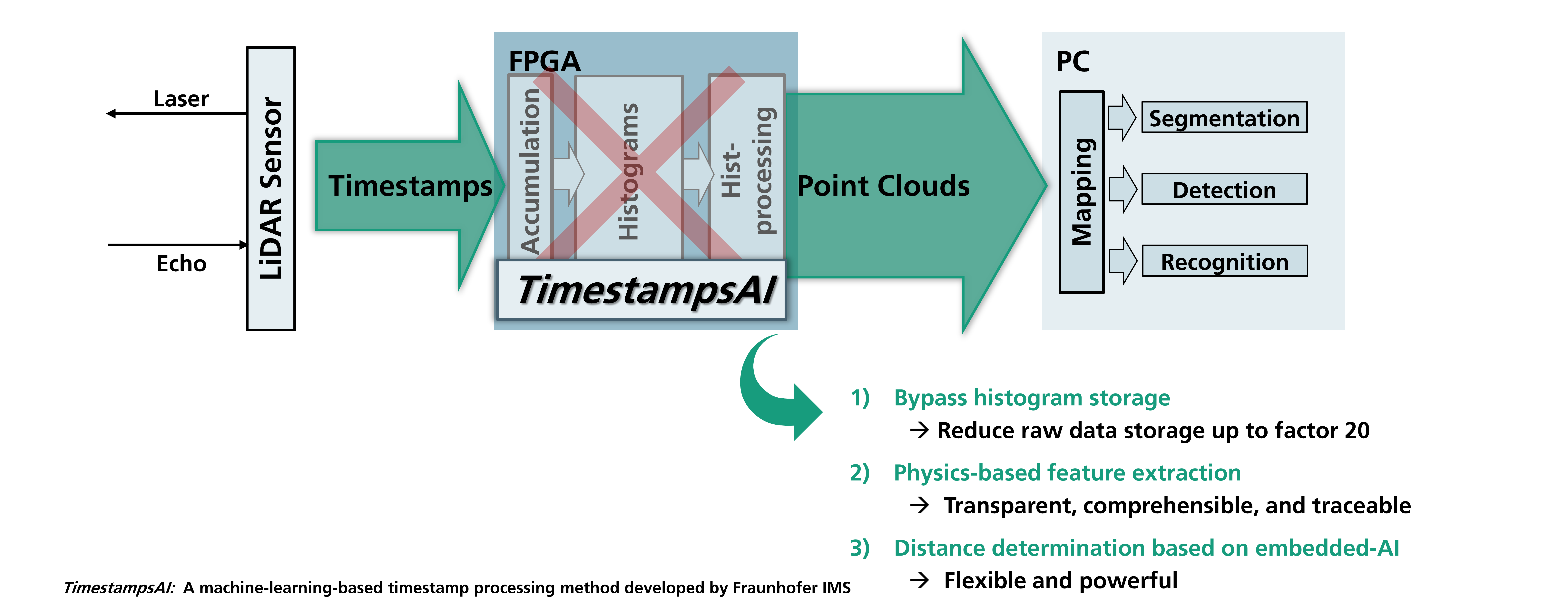